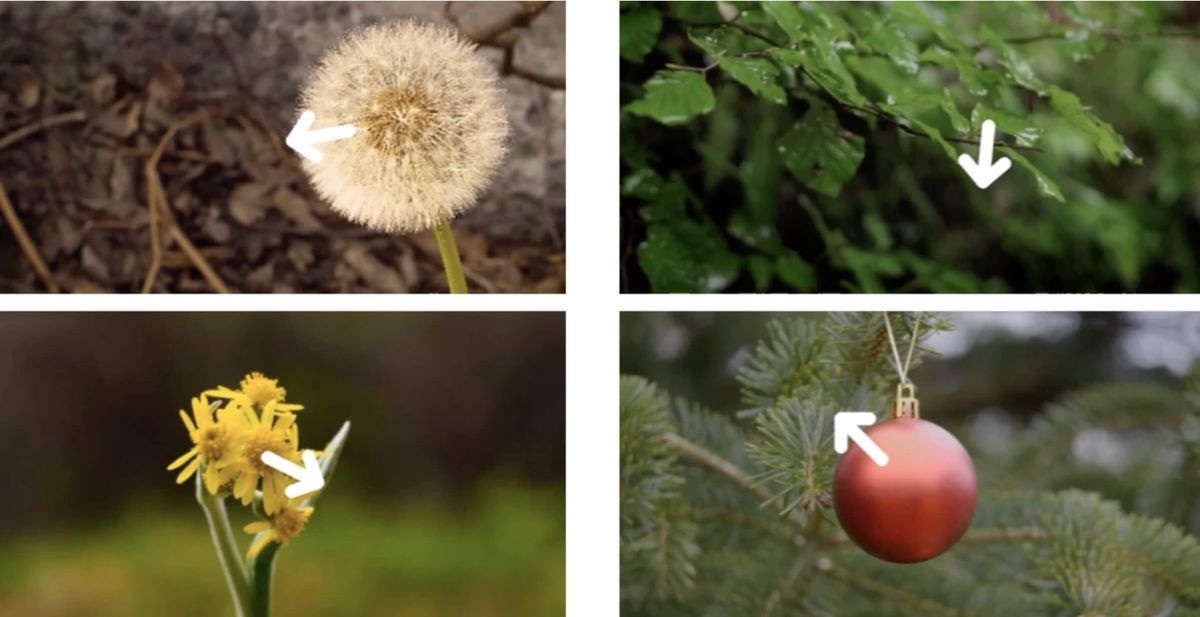
A new study from Google Research demonstrates an AI model that can create realistic motion from a single still image. The model analyzes elements within a photo and can generate a video predicting how these objects would naturally move over time e.g. a swaying tree or flickering candle flame.
In the paper "Generative Image Dynamics", the researchers propose a "neural stochastic motion texture" framework. Given a still image, the system predicts a per-pixel motion spectrum map. This compactly represents plausible oscillating trajectories using a smoothing technique called Fourier basis function. An inverse Fourier transform converts the spectrum into motion by displacing pixels over time according to the predicted motions.
Remarkably, the AI-generated motion is sufficiently accurate to allow for interactive manipulation of the formerly static items, essentially bringing the image to life. This technology signifies a transformational shift in our conception of photographs as frozen moments, turning them into interactive, dynamic windows into plausible realities.
One of the most exciting applications is the image-to-video transformation. By predicting the neural stochastic motion texture for a given static image, the system can create motion that represents natural oscillations like a flower dancing in the breeze, or clothes billowing on a line. This also allows for creative manipulations like slow-motion videos or motion magnification.
The system can also generate videos that loop seamlessly. While seamlessly looping videos are hard to generate traditionally, this research employs a novel motion self-guidance technique to enable smooth transitions between the end and start of a video.
Going beyond traditional video, the model enables interactive dynamics, allowing users to apply forces like poking or pulling to objects within a static image, and observing realistic motion responses. You can play with the demo to see an example. This is made possible by adopting techniques from prior works that simulate object dynamics based on a set of harmonic oscillators.
Compared to previous generative models that animate images by extending them along a time dimension, this approach better captures the underlying physics of natural motions. Qualitative and quantitative experiments demonstrate significant improvements in quality and coherence of generated videos.
This motion prior framework could enable applications like automatically animating photos or creating dynamic AR filters. More broadly, modeling distributions over possible motions, rather than just pixel values, may prove effective for generative modeling of video.
The researchers plan to expand the technique to handle more complex motions beyond oscillations. But for now, this method presents an intriguing new way to bring still images to life.