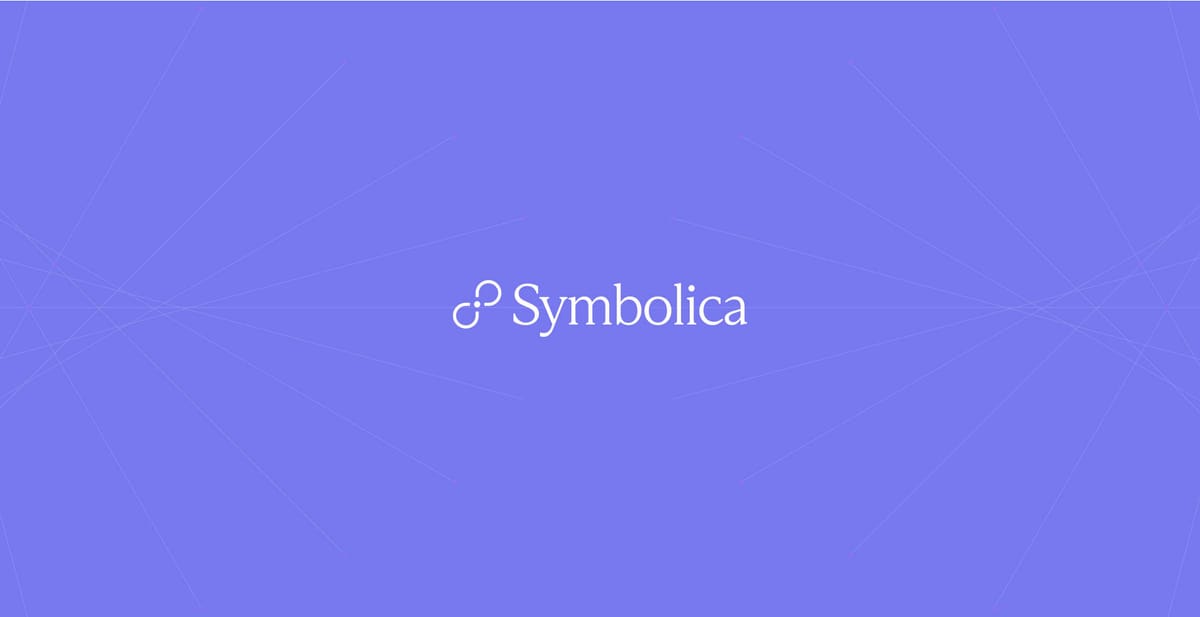
A new startup, Symbolica, is challenging the status quo with a unique approach to building AI models. With $31 million in funding, Symbolica aims to move beyond the limitations of current large language models and create a new era of structured, interpretable, and efficient AI. Founded by former Tesla senior autopilot engineer George Morgan, the startup has raised $31 million in a Series A funding round led by Khosla Ventures, with participation from Day One Ventures, General Catalyst, Abstract Ventures, and Buckley Ventures.
At the core of Symbolica's approach is a powerful mathematical toolkit based on category theory, which enables their models to learn algebraic structure and engage in structured reasoning. This contrasts with the current state-of-the-art large language models like GPT, Claude, and Gemini, which rely on the transformer architecture and are prone to hallucinations and lack interpretability.
By applying category theory, Symbolica aims to develop models capable of structured reasoning. This means that instead of simply predicting the next word, these models will be able to logically process, organize, and generate information based on a set of rules or structured understanding. This structured approach enables interpretability, allowing developers and users to understand and specify how and why the model produces certain outputs.
"What's happening in the industry now is hacks on hacks on hacks," said Morgan in an interview. He believes that the transformer architecture is not the "end-all-be-all" of AI and that Symbolica's framework will enable the development of alternatives that outperform current models.
Vinod Khosla, the lead investor in Symbolica and an early investor in OpenAI, sees great potential in the startup's approach. "We love people coming from left field," Khosla said. He believes that Symbolica offers a "very innovative approach" to one of the biggest challenges facing AI: creating smaller, more efficient models that can reason more like humans.
Symbolica's goal is to move beyond the "alchemy" of contemporary AI and establish a more rigorous, scientific foundation for machine learning. By grounding AI in category theory, the company aims to create models with inherent reasoning capabilities, rather than relying on emergent side effects from training on massive datasets.
This structured approach promises several advantages over current deep learning systems. Symbolica's models are designed to be more interpretable, allowing developers and users to understand and specify how and why outputs were produced. This transparency is crucial for mission-critical applications and regulatory compliance.
Furthermore, by embedding structure directly into the models, Symbolica claims its systems will be significantly more data-efficient than traditional unstructured methods. This means their models can be trained faster, using smaller datasets, with order-of-magnitude improvements in inference speed.
While Symbolica's journey is just beginning, the startup has already garnered attention from the AI research community. The company recently co-authored a paper with Google DeepMind on "categorical deep learning," demonstrating how their approach could supersede previous work on geometric deep learning to build structurally-aware models.
As Symbolica sets its sights on building an entire class of models that outperform the transformer architecture, the road ahead is filled with challenges and opportunities. With its first product, a coding assistant, slated for release in early 2025, the startup will need to prove its theories in practical applications and compete with tech giants investing heavily in AI research and development.
However, if Symbolica succeeds in its mission to bring structured reasoning to AI, the implications could be profound. By moving beyond pattern-matching to genuine machine reasoning, the startup may lay the groundwork for the next great leap forward in artificial intelligence, with applications spanning virtually every industry.
In a landscape where bigger models and more compute power have become the norm, Symbolica is betting that a little structure will go a long way. As Morgan put it, "That's why we can build much smaller models—because we've focused very directly on embedding the structure into the models, rather than relying on large amounts of compute to learn the structure that we could have specified initially."