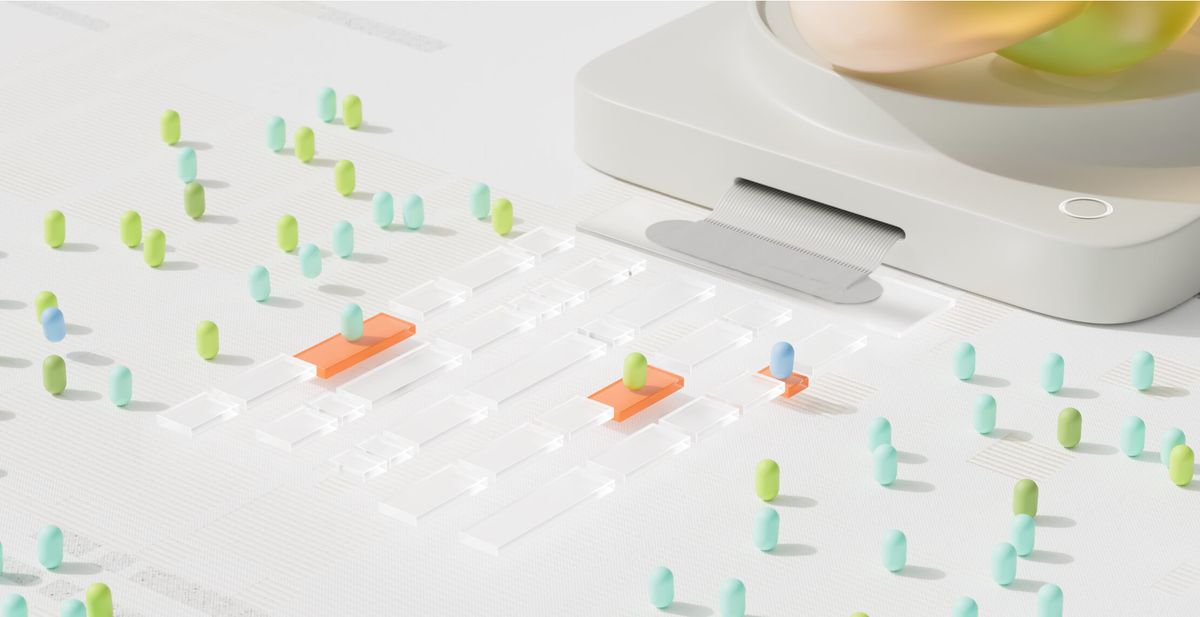
As AI systems grow more advanced, ensuring they are developed and used responsibly has become a pressing concern. AI that can generate misinformation or perpetuate harmful stereotypes, for example, raise ethical questions around how these systems impact society. In a new paper, researchers at Google DeepMind propose a comprehensive framework for evaluating these social and ethical risks of AI systems across different layers of context.
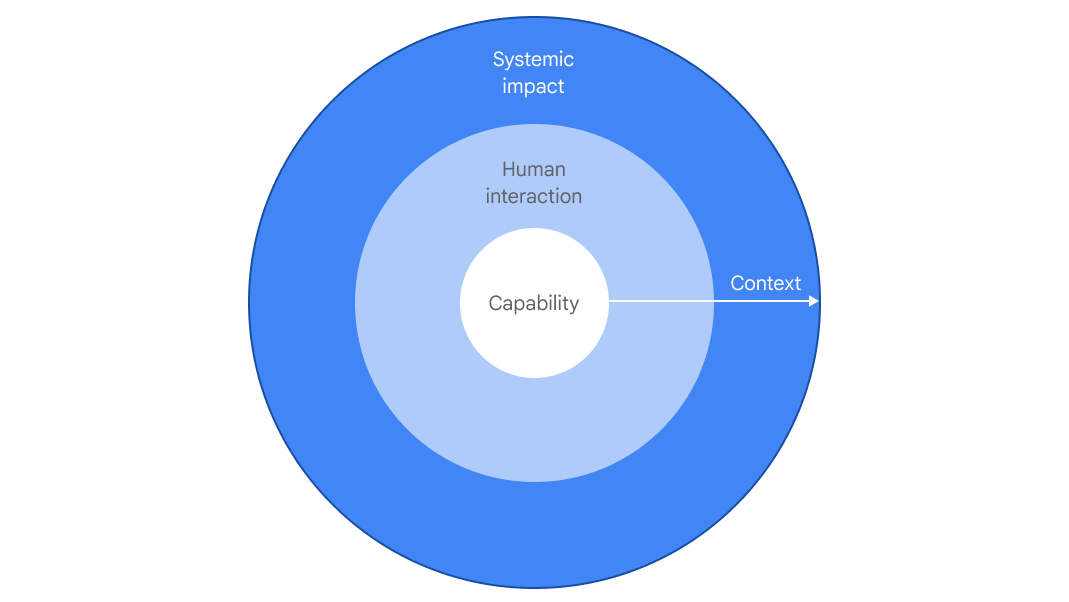
The DeepMind framework evaluates risks at three levels: the system's capabilities, human interactions, and broader systemic impacts. Assessing capability involves testing how likely a system is to produce harmful outputs like incorrect information. But the paper argues even capable systems don't necessarily cause downstream harm without problematic use in context. The next layer evaluates real-world human interactions with an AI system, such as who uses it and whether it functions as intended. Finally, systemic impact assessment analyzes risks that emerge once AI is widely adopted, looking at broad social structures and institutions.
The researchers emphasize that context is critical for evaluating AI risks. Contextual factors weave through each layer of this framework, amplifying the significance of who is using the AI and for what purpose. For instance, while AI might produce factually accurate outputs, its interpretation and subsequent dissemination by users can lead to unforeseen repercussions, which can only be gauged within specific contextual bounds.
Integrating these layers provides a nuanced view of AI safety that goes beyond just model capabilities. The researchers demonstrate this approach through a case study on misinformation. Testing an AI's tendency for factual errors is combined with observing how people use the system and measuring downstream effects like spreading false beliefs. This connects model behavior to actual harm occurring in context, leading to actionable insights.
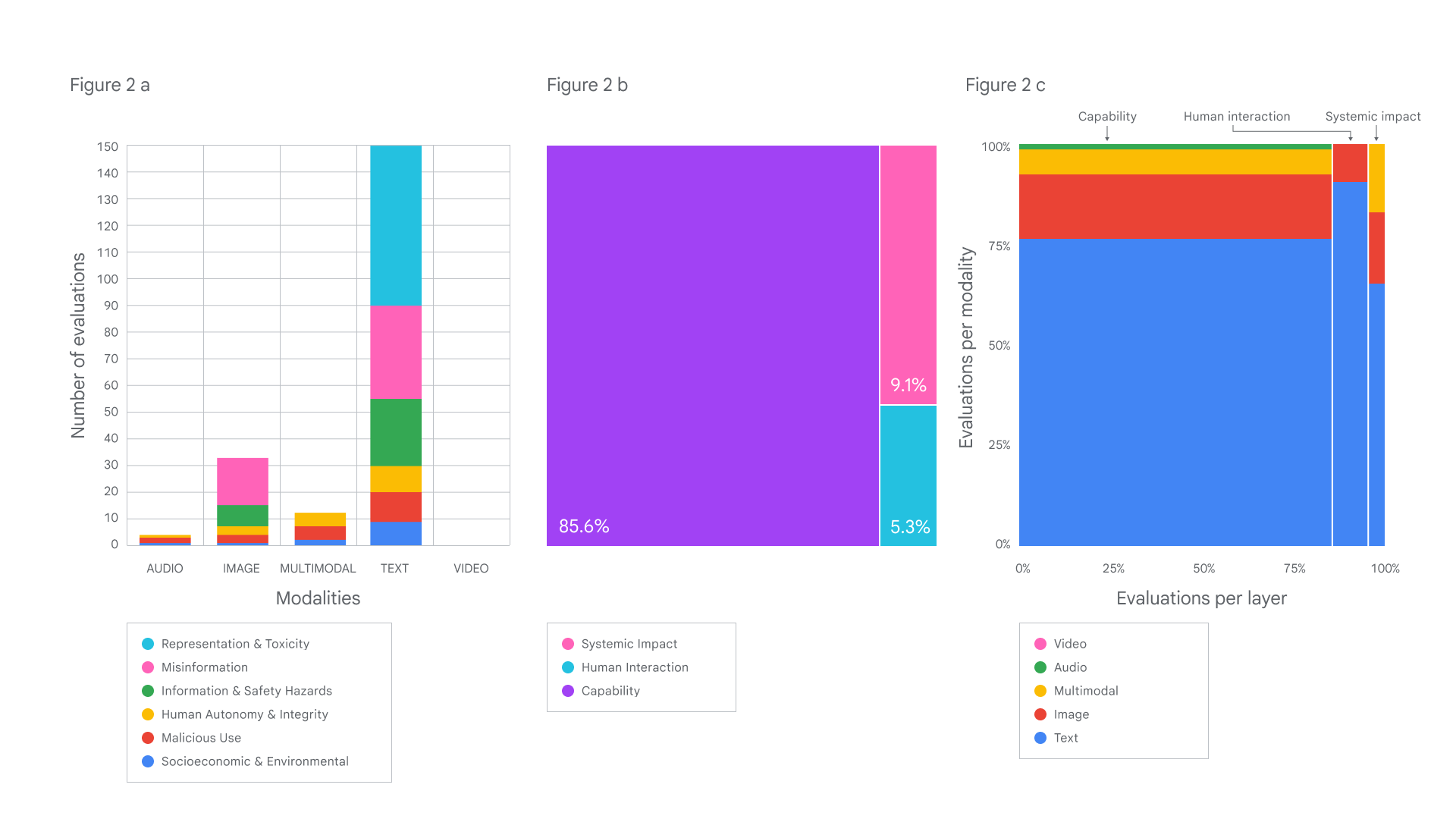
The paper also reviewed current AI safety evaluations and found major gaps. The team emphasized the need to address three areas:
- Context: Existing safety evaluations frequently analyze AI capabilities in a vacuum, often sidelining human interaction and systemic impact points.
- Risk-Specific Evaluations: Current evaluations tend to narrowly define and assess harms, often sidelining nuanced manifestations of risks.
- Multimodality: An overwhelming number of evaluations focus exclusively on text-based outputs. This approach leaves substantial gaps in understanding risks associated with other forms of data, like images, audio, or videos. These different modalities not only enrich the AI experience but also present new avenues for risks to manifest.
The researchers stress AI safety is a shared responsibility. AI developers should interrogate the capabilities of the models they create, while the broader public can assess real-world functionality and societal impacts. Collaboration across sectors is key to robust evaluation ecosystems for safe AI adoption.
As generative AI spreads to domains like medicine and education, rigorous safety assessment is crucial. DeepMind's context-based approach highlights the need to look beyond isolated model metrics. Evaluating how AI systems function in the messy reality of social contexts is essential to unleashing their benefits while minimizing risks. With thoughtful frameworks like these, the AI community can lead development of socially responsible technologies.