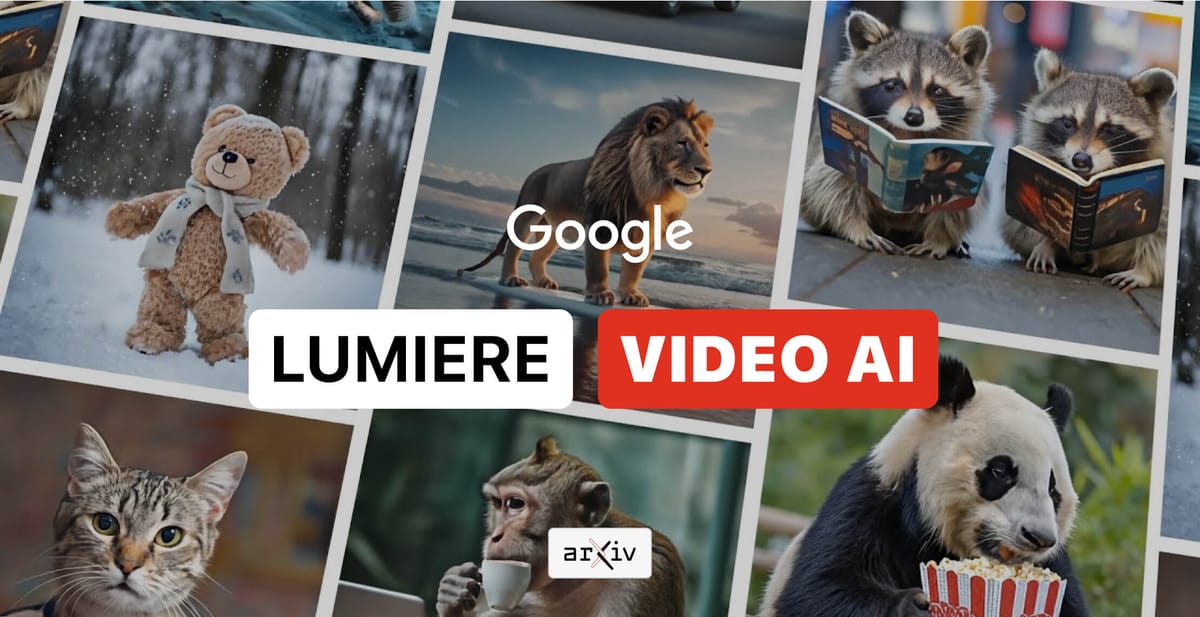
A new research paper from Google AI introduces "Lumiere," a new text-to-video diffusion model, that marks a significant step forward in video synthesis technology. This model is designed to create realistic, diverse, and coherent motion in videos, a task that has historically been challenging in the field of artificial intelligence and computer vision.
Lumiere leverages a novel Space-Time U-Net architecture, a departure from traditional video models. Traditional models generate spatially distant keyframes followed by temporal super-resolution, which often struggles with maintaining global temporal consistency. Lumiere's architecture generates the entire temporal duration of a video in a single pass, enhancing the coherence and fluidity of motion.
Early examples demonstrate remarkably smooth camera movements and intricate object animations spanning multiple seconds. The researchers highlight Lumiere’s suitability for various creative applications beyond text-to-video generation:
Image-to-Video: The model smoothly converts still images into video by conditioning on the first frame.
Video Inpainting: Lumiere can animate arbitrarily masked regions of existing video based on text prompts. This raises intriguing possibilities for video editing, object insertion and/or removal.
Stylized Generation: By combining Lumiere with artistic image priors, the researchers produce eye-catching results transferring spatial styles, like watercolor filters, to temporal video dimensions.
Cinemagraphs: Localized motion effects are possible where part of the image remains static while another part exhibits motion, adding a captivating aesthetic to still images.
The paper also demonstrates directly feeding Lumiere’s outputs into off-the-shelf video filtering techniques to stylize full clips in a temporally consistent manner. This further showcases the versatility of the proposed approach.
According to the researchers, a core limitation of existing cascaded schemes is failing to resolve ambiguous fast motion that becomes temporally aliased when sampled only at sparsely predicted keyframes. Attempting to add motion clarity by interpolating between such frames with temporal super resolution then becomes an uphill battle.
By handling the entire duration directly, Lumiere circumvents such temporal aliasing pitfalls altogether. The results are videos with improved continuity and realism for periodic motions like walking or head turning.
Despite the advancements, Lumiere remains limited when it comes to videos requiring transitions between distinct scenes and shots. This capability gap presents an important direction for future diffusion model research.
Nonetheless, by moving closer to generating intricate object and camera movements in a holistic manner, Lumiere pushes text-to-video generation nearer the cusp of unlocking truly versatile and creative visual synthesis.