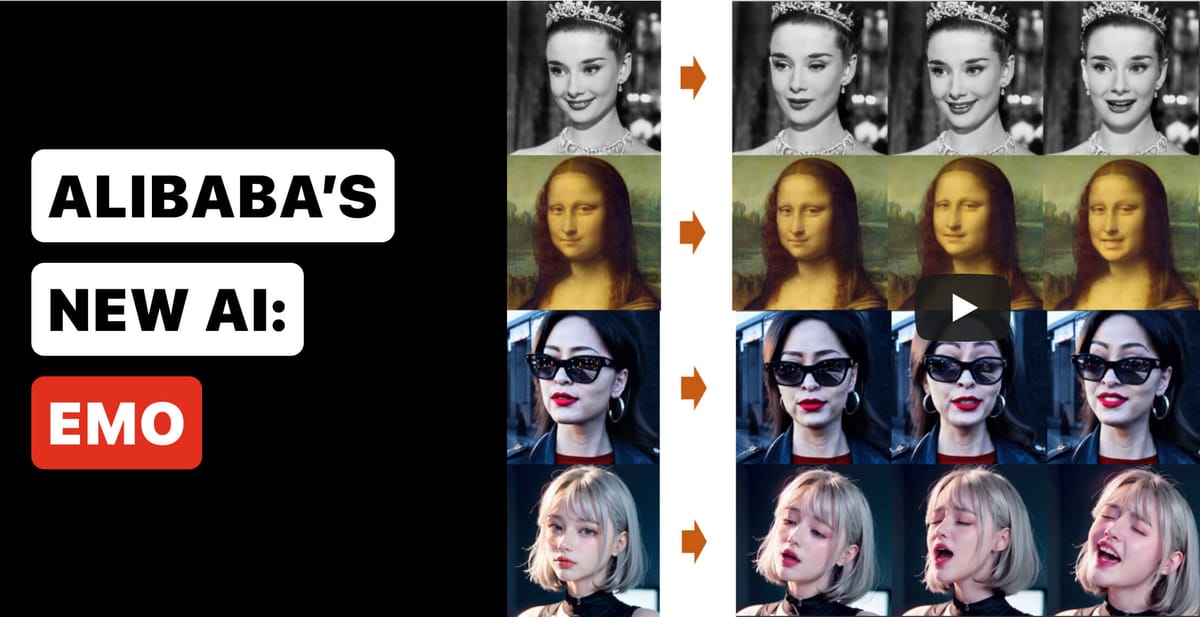
Researchers at Alibaba have introduced EMO, a new AI framework that can generate highly expressive and realistic "talking head" videos from a single reference image and an audio file. It is unbelievable—literally.
Traditional techniques for talking head video generation have often struggled to capture the full range of human expressions and individual facial styles. Methods relying on 3D modeling often fail to capture intricate facial expressions. Direct generation techniques struggle with consistency over time. However, EMO shows that given sufficient data and an appropriate framework, AI can produce remarkably vivid talking head videos that capture nuances of speech.
In a recently published paper, the researchers provide a comprehensive overview of the framework's capabilities. EMO can generate vocal avatar videos with expressive facial expressions and various head poses, all while preserving the character's identity over extended sequences. The output can be any length—it depends on the input audio. This means you can create long-form content with consistent quality.
At EMO's core is a deep neural network leveraging diffusion models - similar to what you have in DALLE or Midjourney. By conditioning these models on audio instead of text or images during training, EMO learns to reverse engineer subtle facial motions precisely matching sounds.
This audio-to-video approach means EMO can animate portraits without predefined animations. An encoder parses acoustic features related to tones, rhythms and emotional affect, driving generation of corresponding mouth shapes and head movements. Concurrently, a reference encoder preserves the visual identity throughout.
Several components work together for fluid, stable videos:
- Temporal modules enable smooth frame transitions and lifelike motions over time by processing groups of frames together.
- A facial region mask focuses detailing on key areas like the mouth, eyes and nose essential for conveying expressions.
- Speed control layers stabilize the pace of head movements across extended sequences to avoid jarring changes.
One of the key strengths of EMO is its ability to generate highly realistic speaking and singing videos in various styles. The system is capable of capturing the subtleties of human speech and song, producing animations that closely resemble natural human movement. This versatility opens up a wide range of potential applications, from entertainment to education and beyond.
In addition to its impressive video generation capabilities, EMO also demonstrates remarkable proficiency in handling diverse portrait styles. The system can animate characters of various styles, including realistic, anime, and 3D, using identical vocal audio inputs. This results in uniform lip synchronization across different styles, further showcasing EMO's adaptability.
Experimental results demonstrate that EMO significantly outperforms existing state-of-the-art methodologies in terms of expressiveness and realism. The system excels in generating lively facial expressions, as evidenced by its superior performance on the Expression-FID metric. Furthermore, EMO's ability to preserve the character's identity over extended sequences is a testament to its robustness and consistency.
Overall, while some limitations around artifacts remain, EMO represents exponential progress in learning to map audio directly to facial motions. It exemplifies AI’s potential to unlock ever greater expressiveness in synthesized human video.