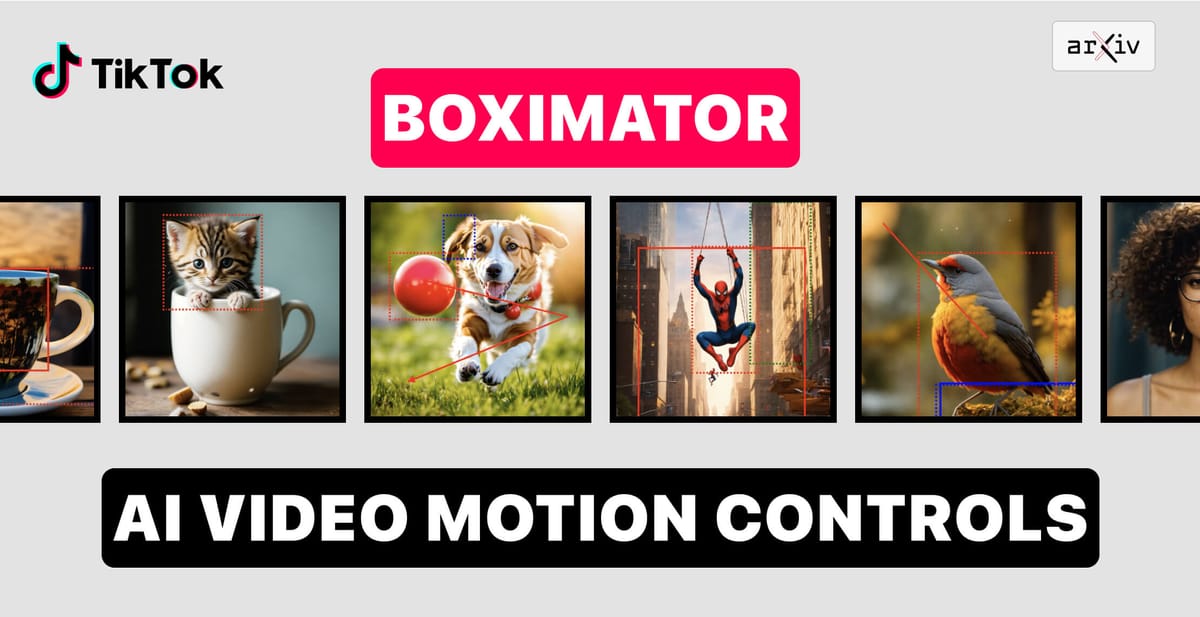
ByteDance, the parent company of TikTok has published a research paper on Boximator, a new technique that allows for remarkably fine-grained control over object motion in generated videos. Take a look:
"The kitten is hiding herself into the cup" | "Spiderman swings towards the camera." | "A woman is running on the street with a dog." | "A boy and a girl are kissing." |
Boximator (a portmanteau of the words "box" and "animator") introduces a simple yet powerful approach for motion specification. Users first select objects in a reference image by drawing boxes around them. They can then define an object's ending position or entire motion path across frames using additional boxes and lines. This visually-grounded technique avoids the need for verbally describing desired motions.
Under the hood, Boximator functions as a plug-in that infuses existing video synthesis models with these user constraints. It trains an additional module while freezing base model weights, enabling straightforward integration with state-of-the-art systems.
Empirically, the Boximator-enhanced models retain the original video quality, measured by Fréchet Video Distance (FVD) scores, while gaining precise motion control capabilities. On the MSR-VTT dataset, the module improved two base models’ FVDs while achieving strong motion alignment, quantified through average precision metrics that compare generated motions against ground truth boxes.
Boximator | Pika 1.0 | Gen-2 |
"A cute 3D boy is standing and then walking." | ||
"Adding wine to a glass." | ||
"The wind blows a woman's umbrella away, rainy day." | ||
Qualitative results further highlight the techniques realism with objects faithfully following complex user-defined paths, interactions, and scene entries/exits. Boximator manages composite elements like a man on a horse, and controls object count, size, proximity, and more.
This marks a significant step towards more versatile video generation platforms that balance quality, diversity, and user control. By externalizing motion specification, Boximator could potentially save substantial compute needed to learn such finer-grained aspects internally.