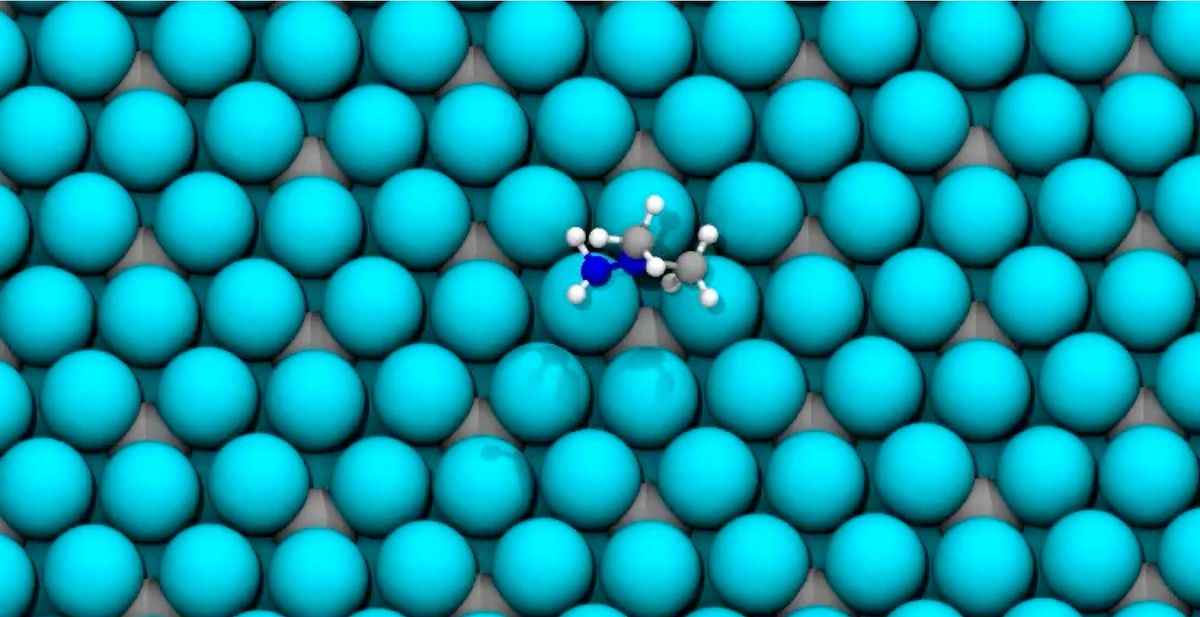
Material sciences could be on the cusp of a major transformation, with Meta's recent release of the Open Catalyst Demo. Their research highlights the power of AI to potentially accelerate discoveries by enabling scientists to model simulations up to 1000 times faster than previous computational methods.
Catalysts are the unsung heroes that quietly power our everyday lives:
- The catalytic converters in automobiles use specialized catalysts to break down harmful exhaust.
- Catalysts transform atmospheric nitrogen into ammonia, a key ingredient in fertilizers that sustain global food production.
- Electrocatalysts split water to produce renewable hydrogen for energy storage. In fuel cells, catalysts combine hydrogen and oxygen to generate electricity for vehicles.
- Carbon capture systems employ catalysts to convert waste and carbon dioxide into useful chemicals.
The development of more efficient, robust, and cost-effective catalysts through material science is therefore indispensable for addressing pressing challenges like climate change and clean energy.
Discovering of these catalysts, however, has traditionally been hindered by slow, labor-intensive physical experiments or computational simulations using Density Functional Theory (DFT).
Meta's Fundamental AI Research (FAIR) in collaboration with Carnegie Mellon University's Department of Chemical Engineering hope to change this with their release of the Open Catalyst Demo. The tool enables researchers to look for optimal binding sites for adsorbate molecules on a catalyst surface. It returns both the adsorption energy and the corresponding structure—crucial outputs for the design of materials with specific properties, such as their ability to catalyze CO2 reduction reactions or green H2 generation.
The demo generates numerous configurations of the adsorbate on the catalyst surface. It then utilizes advanced graph neural networks, developed on Open Catalyst datasets (GemNet-OC and EquiformerV2), to predict energy and force outputs for each configuration. This prediction fuels the process of structure relaxation, replicating the role of DFT but at a dramatically faster pace.
Currently, the demo supports 11,427 catalyst materials and 86 adsorbates. With around 100 surfaces per catalyst and 100 starting configurations for each combination, the platform can computationally explore an astonishing 10 billion structure relaxations—a feat unimaginable with DFT, which takes hours to days for a single relaxation on a GPU compared to just a few minutes with Meta's models.
All the model weights and training/inference codes are publicly available on the ocp repo. The aim is to make the technology available to a broad community of researchers, driving faster and wider exploration of potential new materials.
Meta notes that while the demo uses state-of-the-art models, it has critical limitations compared to DFT that should be considered. E.g., it doesn't account for several important factors in electrochemical devices, like charges, solvents, temperature, surface imperfections, etc. Additionally, the demo may not always find the optimal lowest energy state and researchers are therefore advised to verify predictions experimentally.
Nonetheless, by vastly expanding the horizons of computational exploration, AI looks set to become an indispensable asset in the chemist's toolkit. An asset that could accelerate discoveries and innovations to address climate change.
Despite these constraints, Meta's foray into the material sciences with AI is a promising development. As part of its wider commitment to renewable energy and net-zero carbon emissions, the company understands that catalysts are crucial to the development of more efficient renewable energy and energy storage technologies. Moreover, the underlying graph neural networks that power the Open Catalyst Demo represent an exciting domain in AI research that Meta is keen to explore further.