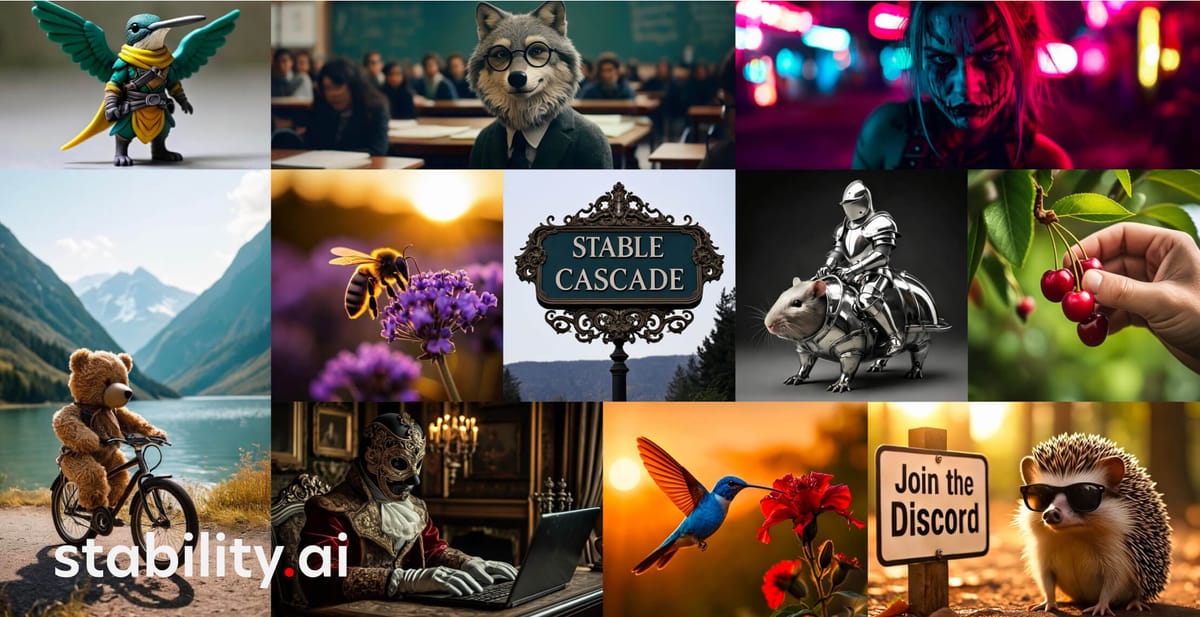
Stability AI has announced the release of Stable Cascade, a new text-to-image architecture focused on remarkable quality, flexibility, and hardware efficiency. Built on a three-stage pipeline of distinct neural networks, Stable Cascade achieves state-of-the-art results while compressing the latent space to enable training and fine-tuning on consumer GPUs. This breakthrough will allow more users than ever before to participate in AI image generation, augmentation, and experimentation.
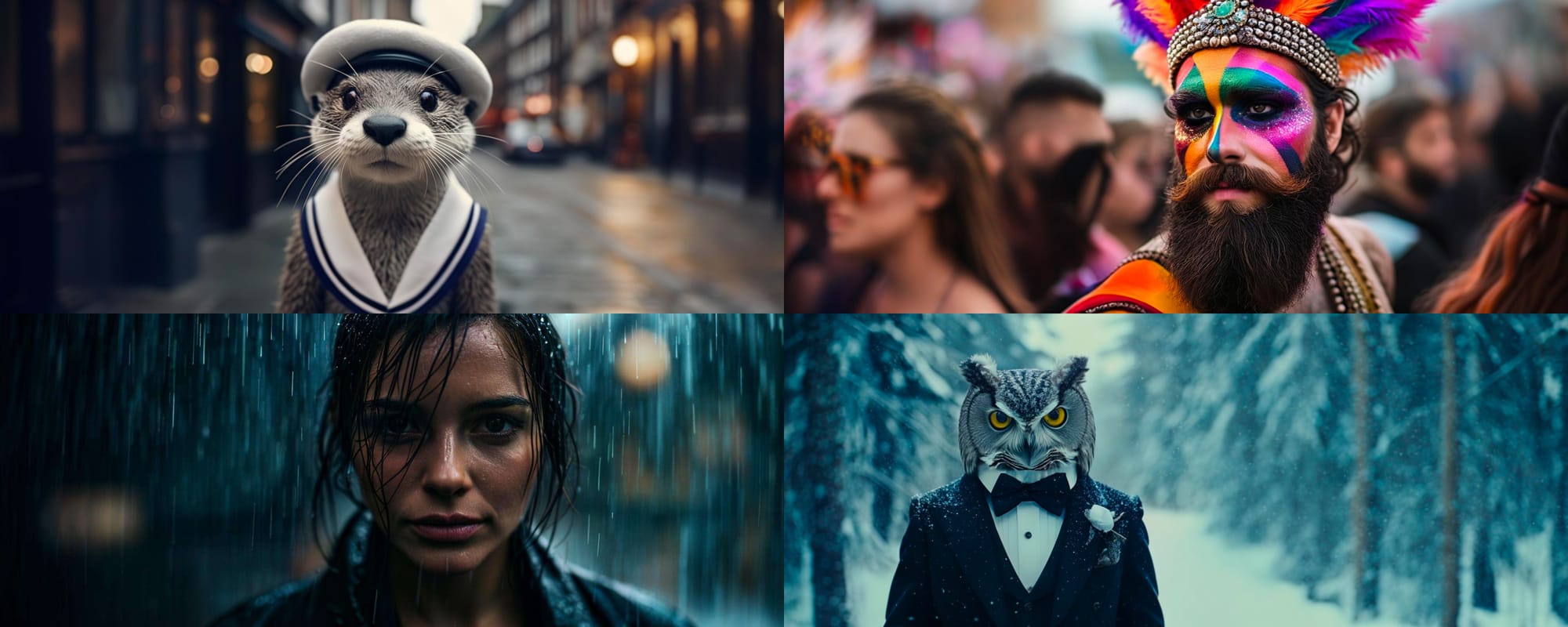
The key innovation enabling Stable Cascade’s capabilities is its compression of the latent space - the abstract representation of an image parsed by the AI. The model consists of three distinct phases: the Latent Generator (Stage C), which converts user inputs into compact 24x24 latents, followed by the Latent Decoder (Stages A & B), tasked with compressing images to achieve unparalleled output quality through a highly compressed latent space.
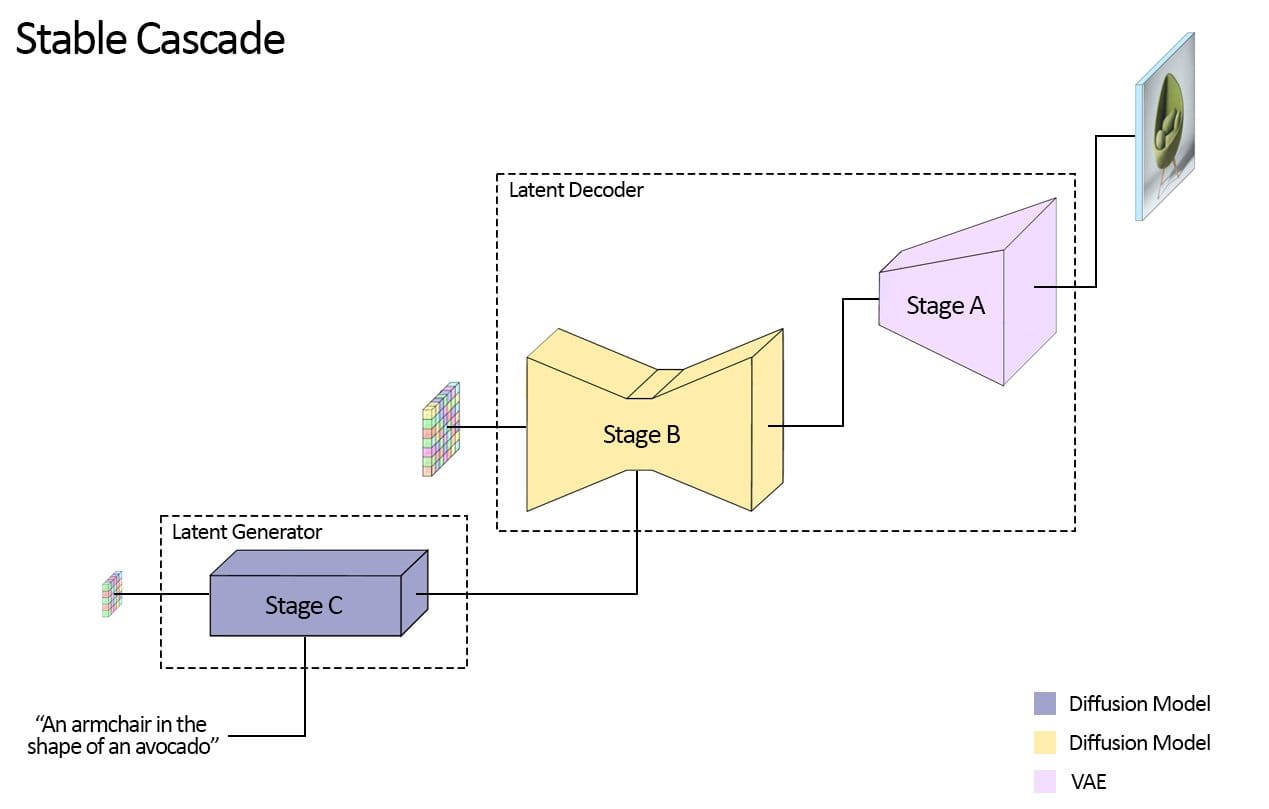
Stable Cascade’s modular design also permits targeted fine-tuning of each stage individually. By decoupling the text-conditional generation from the high-resolution decoding process, Stability AI has achieved a 16x cost reduction in training compared to similar-sized models. This makes the technology not only more affordable but also more adaptable to a wider range of applications. Users are encouraged to focus their efforts on Stage C for most uses, leveraging the provided training scripts, ControlNet, and LoRA training capabilities to experiment with this cutting-edge architecture.
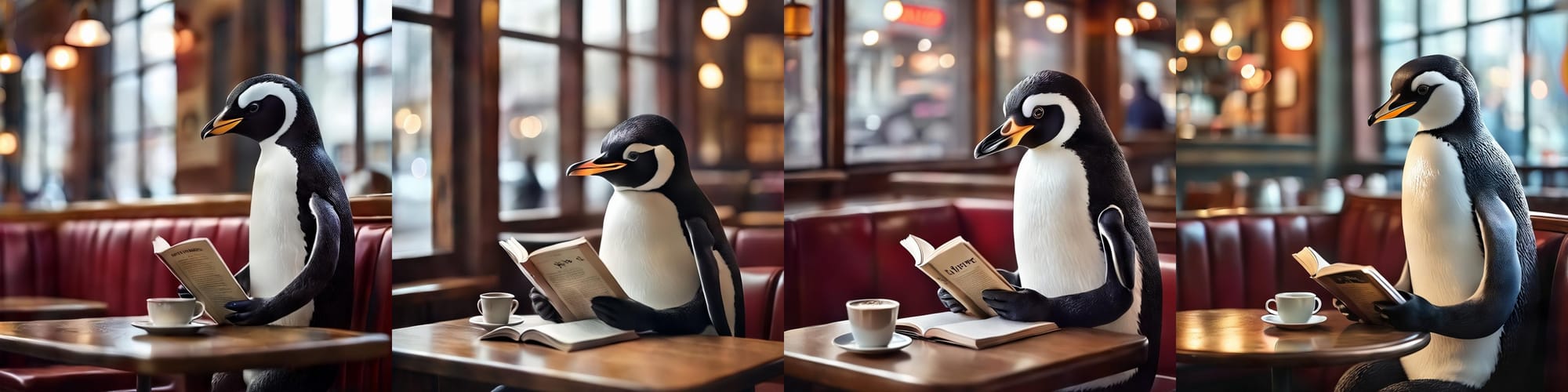
Stable Cascade introduces two models for Stage C (1B & 3.6B parameters) and two for Stage B (700M & 1.5B parameters), with the 3.6B variant of Stage C recommended for those seeking the highest quality outputs. Despite its modular approach, Stable Cascade manages to keep the VRAM requirements for inference remarkably low, around 20gb, further democratizing access to high-fidelity image generation.
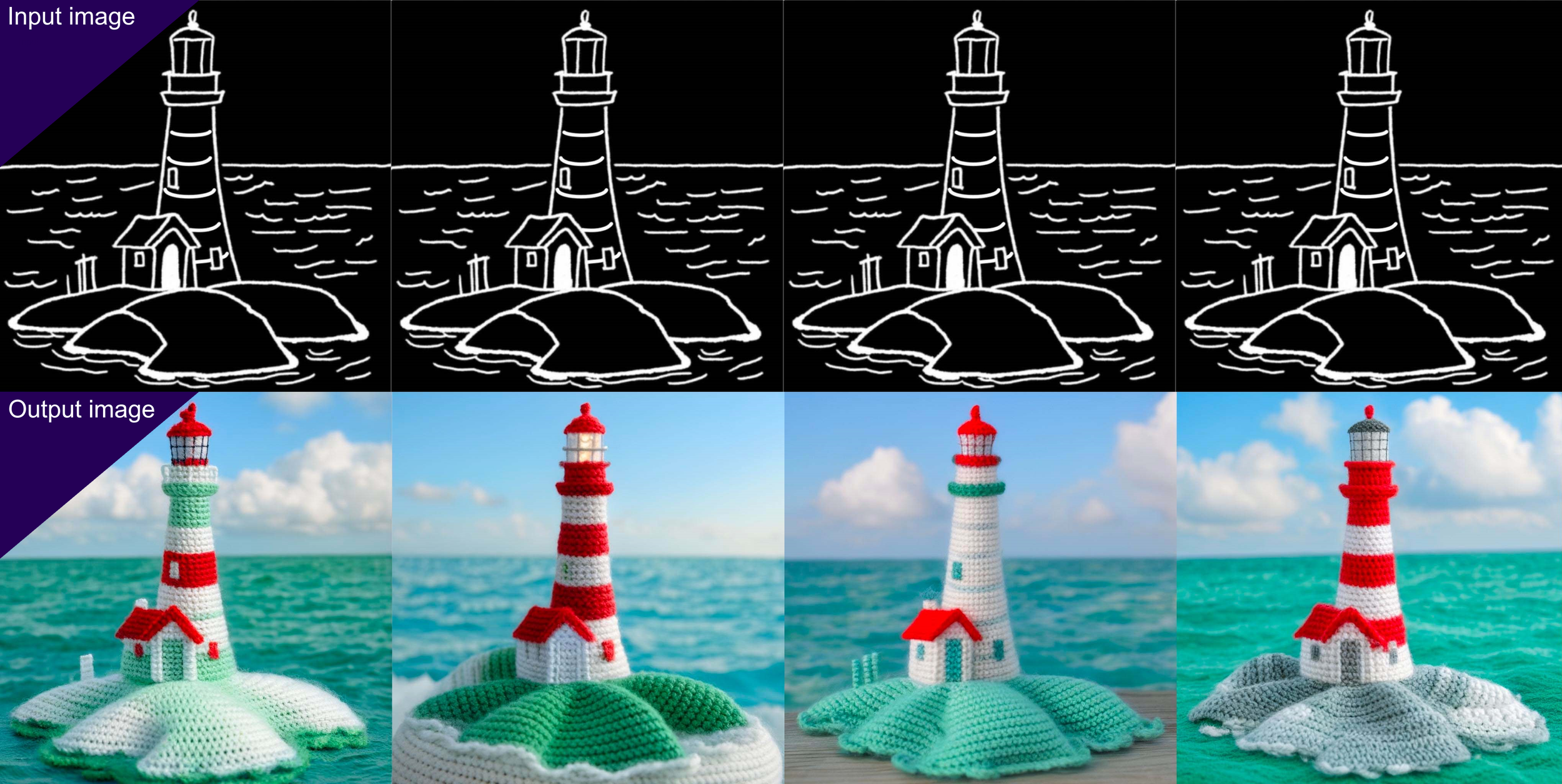
Beyond standard text-to-image generation, Stable Cascade excels in producing image variations and facilitating image-to-image transformations. These features enable users to explore the vast landscapes of their creativity, from generating multiple interpretations of a single image to transforming existing images based on new prompts, illustrating the model's versatility and adaptability.
The company has released all the necessary code for training, fine-tuning, ControlNet, and LoRA on GitHub to empower customization. They have also included scripts for specialized applications such as inpainting/outpainting, canny edge generation, and 2x super-resolution. For now, the model is available only for non-commercial use under strict guidelines pending further policy development.